Using machine learning and mobile phone carrier data to target humanitarian aid
A team of researchers were able to prioritize aid to the poorest people in Togo using machine learning algorithms trained on patterns of mobile phone usage
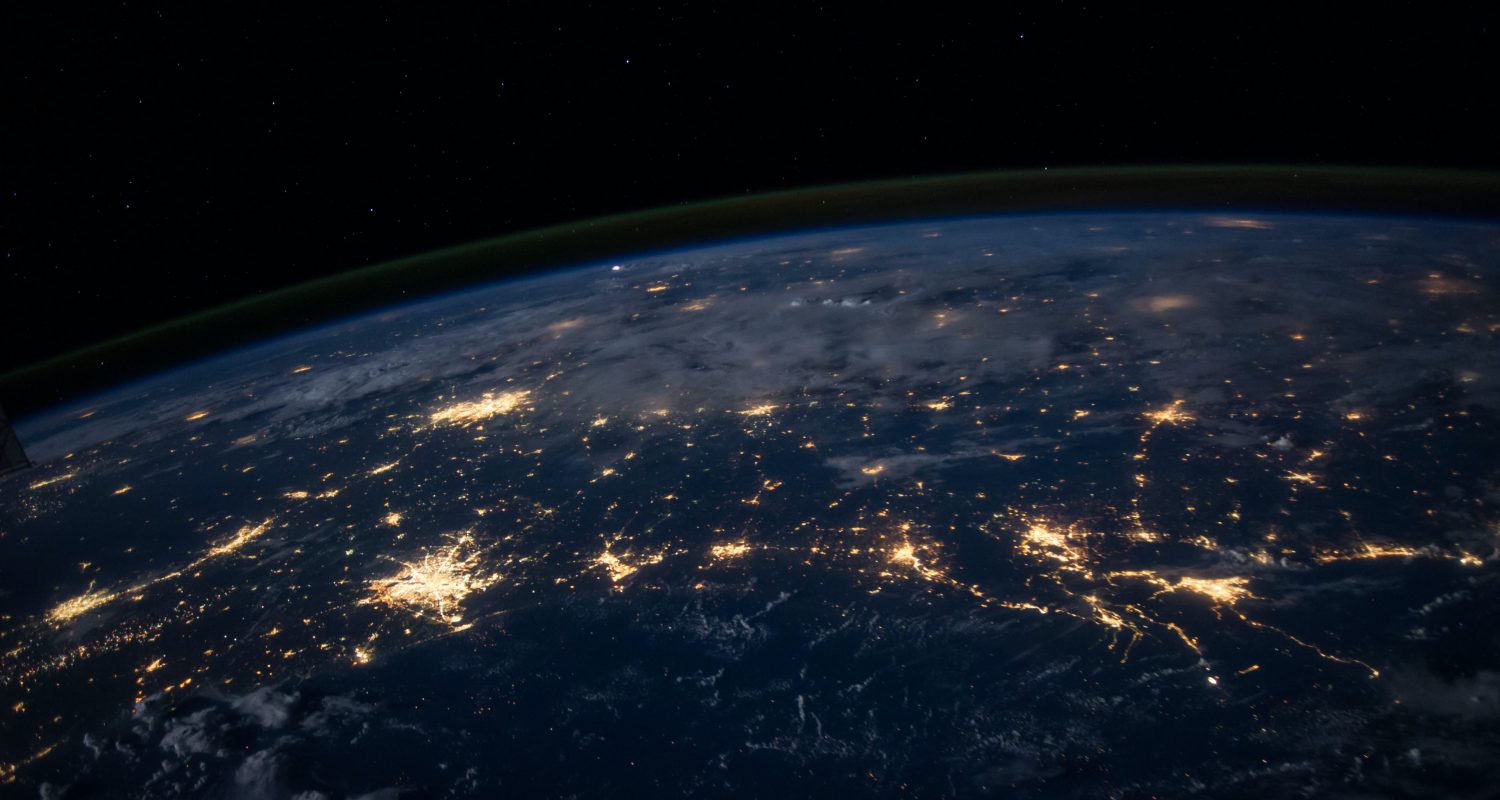
COVID-19 has devastated many low and middle-income countries, causing food shortages and a sharp decline in living standards. In response to this, governments and humanitarian organizations all over the world have distributed social assistance programs to more than 1.5 billion people (Gentilini et al., 2022).
But knowing who to target when administering these programs has become a difficult challenge. How can one quickly identify who needs help the most?
A team of researchers from UC Berkeley, the University of Mannheim, and Northwestern University used survey data, satellite images, and mobile phone networks to train machine-learning algorithms to recognize patterns of poverty in mobile phone data.
By obtaining estimates of the relative wealth of every 2.4 km by 2.4 km region in Togo, constructed by applying machine-learning algorithms to high-resolution satellite imagery, they were able to provide an indication of the relative wealth of all the households in each small grid cell.
In a second step, they estimated the average daily consumption of each mobile phone subscriber by applying algorithms to mobile phone metadata provided by Togo’s two mobile phone operators.
Finally, they conducted surveys with a representative sample of mobile phone subscribers and used such surveys to measure the wealth and consumption of each subscriber, and then matched the survey-based estimates to detailed metadata on each subscriber’s history of phone use. This sample was then used to train supervised machine-learning algorithms that predict wealth and consumption from phone use.
Improvements in targeting performance for the distribution of support and aid programs can translate to an increase in social welfare, especially among the people that need it the most. Nonetheless, there is growing concern that algorithmic decision-making can unfairly discriminate against vulnerable groups.
To read the full article: https://oa.mg/work/10.1038/s41586-022-04484-9
The code used for these analyses is also publicly available at: https://github.com/emilylaiken/togo-targeting-replication/
References
Aiken, E., Bellue, S., Karlan, D., Udry, C., & Blumenstock, J. E. (2022). Machine learning and phone data can improve targeting of humanitarian aid. Nature, 603(7903), 864–870. https://oa.mg/work/10.1038/s41586-022-04484-9
Gentilini, U., Almenfi, M., Okamura, Y., & Dale, P. (2022). Social Protection and Jobs Responses to COVID-19: A Real-Time Review of Country Measures.